See what they’re saying
Generative AI and LLMs at the Edge are key to intelligent situational awareness in verticals from manufacturing to healthcare to defense.Disruptive innovation like BrainChip TENNs support Vision Transformers built on the foundation of neuromorphic principles, can deliver compelling solutions in ultra-low power, small form factor devices at the Edge, without compromising accuracy.
Multimodal Edge AI is an irreversible trend, and it is intensifying the demands on intelligent compute required from Edge devices. We’re excited that the 2nd Generation Akida addresses the critical elements of performance, efficiency, accuracy, and reliability needed to accelerate this transition. Most importantly, BrainChip has been a strategic partner that has collaborated closely with Edge Impulse to make their solutions easy to integrate, develop and deploy to the market.
This is a significant step in BrainChip’s vision to bring unprecedented AI processing power to Edge devices, untethered from the cloud. With Akida’s 2nd generation in advanced engagements with target customers, and MetaTF enabling early evaluation for a broader market, we are excited to accelerate the market towards the promise of Edge AI.
At Prophesee, we are driven by the pursuit of groundbreaking innovation addressing event-based vision solutions. Combining our highly efficient neuromorphic-enabled Metavision sensing approach with Brainchip’s Akida neuromorphic processor holds great potential for developers of high-performance, low-power Edge AI applications. We value our partnership with BrainChip and look forward to getting started with their 2nd generation Akida platform, supporting vision transformers and TENNs.
BrainChip and its unique digital neuromorphic IP have been part of IFS’ Accelerator IP Alliance ecosystem since 2022. We are keen to see how the capabilities in Akida’s latest generation offerings enable more compelling AI use cases at the edge.
BrainChip has some exciting upcoming news and developments underway. Their 2nd generation Akida platform provides direct support for the intelligence chip market, which is exploding. IoT market opportunities are driving rapid change in our global technology ecosystem, and BrainChip will help us get there.
Integration of AI Accelerators, such as BrainChip’s Akida technology, has application for high-performance RF, including spectrum monitoring, low-latency links, distributed networking, AESA radar, and 5G base stations. A leader in small form factor, low power SDR technology.
Through our collaboration with BrainChip, we are enabling the combination of SiFive’s RISC-V processor IP portfolio and BrainChip’s 2nd generation Akida neuromorophic IP to provide a power-efficient, high capability solution for AI processing on the Edge. Deeply embedded applications can benefit from the combination of compact SiFive Essential™ processors with BrainChip’s Akida-E, efficient processors; more complex applications including object detection, robotics, and more can take advantage of SiFive X280 Intelligence™ AI Dataflow Processors tightly integrated with BrainChip’s Akida-S or Akida-P neural processors.
Edge Impulse is thrilled to collaborate with BrainChip and harness their groundbreaking neuromorphic technology. Akida’s 2nd generation platform adds TENNs and Vision Transformers to a strong neuromorphic foundation. That’s going to accelerate the demand for intelligent solutions. Our growing partnership is a testament to the immense potential of combining Edge Impulse’s advanced machine learning capabilities with BrainChip’s innovative approach to computing. Together, we’re forging a path toward a more intelligent and efficient future.
Ai Labs is excited about the introduction of BrainChip’s 2nd generation Akida neuromorphic IP, which will support vision transformers and TENNs. This will enable high-end vision and multi-sensory capability devices to scale rapidly. Together, Ai Labs and BrainChip will support our customers’ needs to address complex problems. Improving development and deployment for industries such as manufacturing, oil and gas, power generation, and water treatment, preventing costly failures and reducing machine downtime.
We see an increasing demand for real-time, on-device, intelligence in AI applications powered by our MCUs and the need to make sensors smarter for industrial and IoT devices. We licensed Akida neural processors because of their unique neuromorphic approach to bring hyper-efficient acceleration for today’s mainstream AI models at the edge. With the addition of advanced temporal convolution and vision transformers, we can see how low-power MCUs can revolutionize vision, perception, and predictive applications in a wide variety of markets like industrial and consumer IoT and personalized healthcare, just to name a few.
We see a growing number of predictive industrial (including HVAC, motor control) or automotive (including fleet maintenance), building automation, remote digital health equipment and other AIoT applications use complex models with minimal impact to product BOM and need faster real-time performance at the Edge. BrainChip’s ability to efficiently handle streaming high frequency signal data, vision, and other advanced models at the edge can radically improve scale and timely delivery of intelligent services.
Advancements in AI require parallel advancements in on-device learning capabilities while simultaneously overcoming the challenges of efficiency, scalability, and latency. BrainChip has demonstrated the ability to create a truly intelligent edge with Akida and moves the needle even more, in terms of how Edge AI solutions are developed and deployed. The benefits of on-chip AI from a performance and cost perspective are hard to deny.
BrainChip’s cutting-edge neuromorphic technology is paving the way for the future of artificial intelligence, and Drexel University recognizes its immense potential to revolutionize numerous industries. We have experienced that neuromorphic compute is easy to use and addresses real-world applications today. We are proud to partner with BrainChip and advancing their groundbreaking technology, including TENNS and how it handles time series data, which is the basis to address a lot of complex problems and unlocking its full potential for the betterment of society.
Key Benefits
2nd Generation akidaTM expands the benefits of Event-Based, Neuromorphic Processing to a much broader set of complex network models.
It builds on the Technology Foundations, adds 8 bit weights and activations support and key new features that improve energy efficiency, performance and accuracy, while minimizing model storage needed, thereby enabling a much wider set of Intelligent applications that can be run on Edge devices untethered from the cloud.
akidaTM 2nd Generation Platform Brief
Engaging with lead adopters now. General availability in Q3’2023.
Explore new 2nd Generation Features
Which efficiently accelerate Vision, Multi-Sensory and Spatio-Temporal applications such as
- Audio Processing, Filtering, Denoising for Hearing Aids, and Hearables
- Speech Processing for Consumer, Automotive and Industrial Applications
- Vital Signs Prediction & Monitoring in Healthcare
- Time Series Forecasting for Industrial Predictive Maintenance
- Video Object Detection and Tracking in Consumer, Automotive and Industrial
- Vision, LIDAR Analysis for ADAS
- Advanced Sequence Prediction for Robotic Navigation and Autonomy
These capabilities are critically needed in Industrial, Automotive, Digital Health, Smart Home, and Smart City Applications.
On-Chip
Learning
Eliminates Sensitive Data
sent to Cloud and Improves
Security & Privacy.
Multi-Pass
Processing
Supports 1 To 128 Node
Implementations and
Scales Processing.
Configurable
IP Platform
Flexible and Scalable
for Multiple Edge
AI Use Cases.

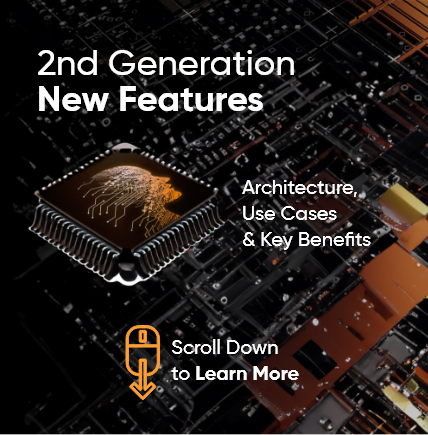
Temporal Event-Based
Neural Nets
Neural Nets
Definition
A patent-pending innovation that enable an order of magnitude, or more, reduction in model size and resulting computation for Spatio-Temporal Applications while maintaining or improving accuracy.
TENNs are easily trained with back-propagation like a CNN and inference like a RNN. They efficiently process a stream of 2D frames or a stream of 1D values through time.
Key Benefits:
- Reduced Footprint & Power: TENNs radically reduces the number of parameters and resulting computation by orders of magnitude.
- High Accuracy & Speed: Reduced footprint does not affect accuracy of results. Lesser computation needed results in greater speed of execution.
- Simplified Training: Pipeline similar to CNN training, but with benefits of RNN operation.
Visualization
The spatial and temporal aspects of the data seamlessly processed by TENNs. In this case, the input data is a stream of 2D video frames processed through time.
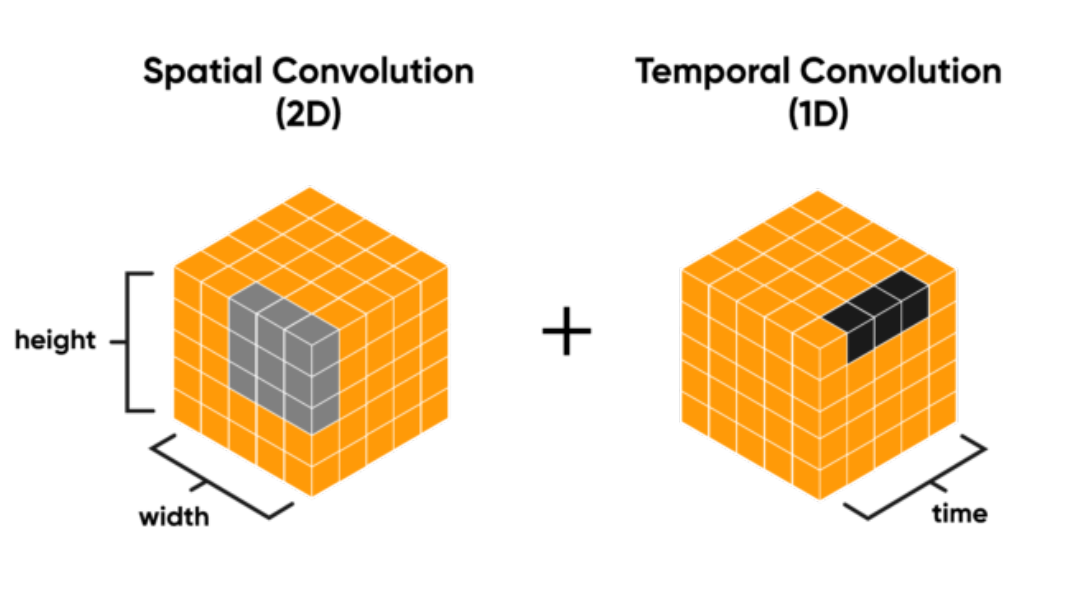
Popular for tasks like
Real-Time Robotics
Quickly processing environmental changes for drone navigation or robotic arms.
Early Seizure Detection
Quickly detect and predict the onset of epileptic seizures in real-time.
Dynamic Vision Sensing
Fast motion tracking and optical flow estimation in changing visual scenes.
Vision
Transformers
Transformers
Definition
Vision Transformers, or ViTs, are a type of deep learning model that uses self-attention mechanisms to process visual data.
ViTs were introduced in a paper by Dosovitskiy in 2020 and have since gained popularity in computer vision research.
In a Vision Transformer, an image is first divided into patches, which are then flattened and fed into a multi-layer transformer network. A multi-head self-attention mechanism in each transformer layer allows the model to focus on the relationships between image patches at differing levels of abstraction to capture local and global features.
The transformer’s output is passed through a final classification layer to obtain the predicted class label.
Key Benefits:
- Complete hardware acceleration: ViT encoder block fully implemented in hardware for execution independent of host CPU.
- Highly Efficient Performance: Execution in hardware without CPU overhead, highly reduces energy consumption while improving speed.
- Low footprint, High scalability: Compact design reduces overhead. Can scale throughput and capacity with up to 12 nodes.
Visualization
Vision Transformers are like teaching a computer to see by breaking down pictures into small pieces, similar to how you’d piece together a jigsaw puzzle.
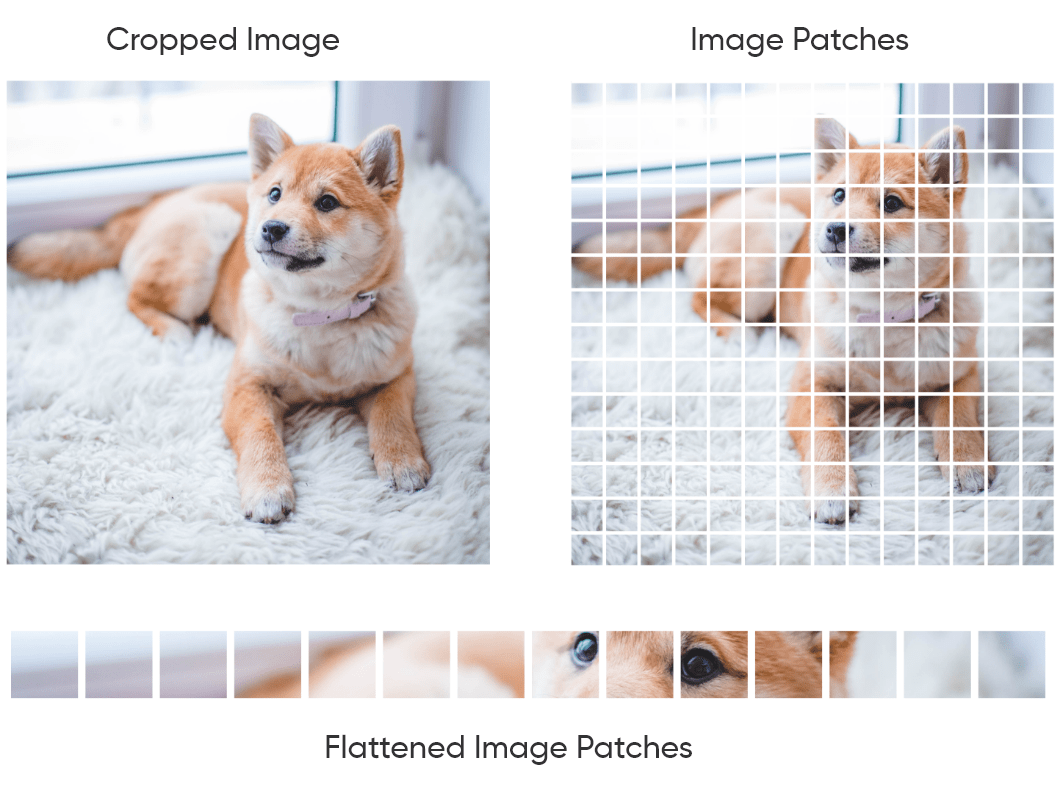
Instead of analyzing the whole image at once, the computer examines each piece and then combines what it learns from all the pieces to understand the entire picture.
Popular for tasks like
Image Classification
Achieving state-of-the-art results on benchmarks like ImageNet.
Object Detection
Detect and classify multiple objects within an image simultaneously.
Image Generation
Producing new images or enhancing existing ones based on learned patterns.
Skip
Connections
Connections
Definition
The foundational akidaTM technology simultaneously accelerates multiple feed-forward networks completely in hardware.
With the added support for short and long-range skip connections, an akidaTM neural processor can now accelerate complex neural networks such as ResNet completely in hardware without model computations on a host CPU.
Skip connections are impemented by storing data from previous layers in the akidaTM mesh or in a scratchpad memory for combination with data in later layers.
Key Benefits:
- Complex Network Acceleration: Enables complete hardware execution of non feed-forward model architectures such as ResNet and DenseNet.
- Low Latency: Eliminates CPU interaction during network evaluation which minimizes model latency.
Visualization
Skip connections effectively allow a neural network to remember the outputs of earlier layers in the network for use in computation in later layers of the network.
This facilitates combining information from different abstraction levels.
Popular for tasks like
Image Segmentation
Help retain details, crucial for precise tasks like medical imaging.
Residual Learning
Allow training deeper layers without degradation, thereby improving overall model performance.
Object Detection
Combine features to detect objects of varying sizes effectively.
Efficiency
Ideal for always-on, energy-sipping Sensor Applications:
Vibration Detection
Anomaly Detection
Keyword Spotting
Sensor Fusion
Low-Res Presence Detection
Gesture Detection
@Sensor Inference
Either Standalone or with Min-spec MCU.
Configurable to ideal fit:
1 – 2 nodes (4 NPE/node)
Anomaly Detection
Keyword Spotting
Expected implementations:
50 MHz – 200
MHz Up to 100 GOPs
Additional Benefits
- Eliminates need for CPU intervention
- Fully accelerates most feed-forward networks
- Optional skip connection and TENNs support for more complex networks
- Completely customizable to fit very constrained power, thermal, and silicon area budgets
- Enables energy-harvesting and multi-year battery life applications, sub milli-watt sensors
Balanced
Accelerates in hardware most Neural Network Functions:
Advanced Keyword Spotting
Sensor Fusion
Low-Res Presence Detection
Gesture Detection & Recognition
Object Classification
Biometric Recognition
Advanced Speech Recognition
Object Detection & Semantic Segmentation
and Application SoCs
With Min-Spec or Mid-Spec MCU.
Configurable to ideal fit:
3 – 8 nodes (4 NPE/node) 25 KB
100 KB per NPE
Process, physical IP and other optimizations
Expected implementations:
100 – 500 MHz
Up to 1 TOP
Additional Benefits
- CPU is free for most non-NN compute
- CPU runs application with minimal NN-management
- Completely customizable to fit very constrained power, thermal and silicon area budgets
- Enables intelligent, learning-enabled MCUs and SoCs consuming tens to hundreds of milliwatts or less
Performance
Detection, Classification, Segmentation, Tracking, and ViT:
Gesture Detection
Object Classification
Advanced Speech Recognition
Object Detection & Semantic Segmentation
Advanced Sequence Prediction
Video Object Detection & Tracking
Vision Transformer Networks
in a Sensor-Edge Power Envelope
With Mid-Spec MCU or Mid-Spec MPU.
Configurable to ideal fit:
8 – 256 nodes (4 NPE/node) + optional Vision Transformer
100 KB per NPE
Process, physical IP and other optimizations
Expected implementations:
800 MHz – 2 GHz
Up to 131 TOPs
Additional Benefits
- CPU is free for most non-NN compute
- CPU runs application with minimal NN-management
- Builds on Performance product with Vision transformer capability
- akidaTM accelerates most complex spatio-temporal and Vision Transformer networks in hardware